MLOps - WorkFlow2: MLFlow 실습
안녕하세요, 이번 포스팅에서는 이전 포스팅 마지막에 언급드렸던 실습에 대해 다뤄보려 합니다!
1. Sklearn을 이용한 MLFlow
0. Background
- Model Train 및 Tracking을 실행하는 방식은 2가지 입니다.
- python 파일을 직접 실행
- ML Project를 이용한 mlflow cli 사용
- 이 글을 작성할 당시에 사용된 특정 모듈에 대한 버전은 다음과 같습니다.
mlflow==2.7.1 bentoml==1.1.5
- required files
MLProject
requirements.txt
python_env.yaml
mlflow_tracking.py
inferences_test.py
product_download.py
train.csv
test.csv
1. 머신러닝 모델 훈련 예시
1. Python 파일 직접 실행
- 파이썬 가상환경에 실행에 필요한 모든 파일들을 설치
pip install -r requirements.txt
- 파이썬 파일 실행
python mlflow_tracking.py
- 파일을 실행하면
mlruns
라는 폴더가 생기면서 0 폴더가 생깁니다.0 = Default
- 모델과 데이터를 저장한 것들은
artifacts
에 저장 됩니다. - 실험 결과를 측정하는 값은
metrics
, 로깅하고 싶은 파라미터 혹은 실험 값들은params
폴더에 저장됩니다.
- 파일을 실행하면
- MLFlow 의 web ui 시작
mlflow server --host=0.0.0.0 --port=5000
- 웹사이트 접속 시, 가장 먼저 보이는 ui 입니다.
- 왼쪽 탭에선 수행한 실험들을 고를 수 있습니다.
- 수행된 실험을 누르면 해당 실험에 속해있는 run 요소들을 볼 수 있습니다.
- run 을 클릭하면 앞서 본 것과 같은 run_id 를 가지고 있음을 확인이 가능합니다.
- parameters, metric 에는 각각
log_param
,log_metric
으로 정의한 값들이 들어가 있습니다.
artifact
에는 실제 모델에 관한 정보들이 담겨 있습니다.
- 한번 더 실험을 수행
💡MLFlow에는 실험이라는 개념이 있고, 각 실험은 여러번의 실행을 가질 수 있습니다.
- 훈련된 모델로 추론해보기.
python inferences_test.py
# inferences_test.py
import mlflow
import pandas as pd
if __name__ == "__main__":
# runs:/{run_id}/{실행폴더}
logged_model = "runs:/6be888244717458c8fafe6f255eea627/titanic-model"
loaded_model = mlflow.pyfunc.load_model(logged_model)
test_x = pd.DataFrame(
{
"Pclass": [2, 1],
"Sex": [0, 1],
"Fare": [3.3211, 3.3211],
"SibSp": [3, 3],
"Parch": [3, 3],
}
)
print(loaded_model.predict(test_x))
2. MLProject를 사용한 mlflow run
- MLProject 파일 생성
# MLProject
name: MlFlow-Tutorial
python_env: python_env.yaml
entry_points:
main:
parameters:
model-name: { type: string, default: "titanic-model" }
command: "python mlflow_tracking.py -m {model-name}"
- 실험을 수행할 경로에 MLProject 의 이름으로 파일을 생성
- 실험을 위해 직접 넣어주던 명령어를 그대로 수행할 수 있는 템플릿을 만드는 것
mlflow run
을 통한 실행mlflow run . --env-manager=virtualenv --experiment-name=titanic-project --run-name=titanic-project-1
- —env-manager: virtualenv 의 가상환경으로 실행한다는 의미
- —experiment-name: 실험의 이름을 지정
- —run-name: run의 이름을 지정
→ 앞서 생성한 방식과 마찬가지로 Experiment와 Run 이 생성됩니다.
- (extra) 단,
env_manager
와 관련해서 추가 설정이 필요합니다.- Local 환경으로 실행일 경우
- 로컬에서 생성한 가상환경으로 실행하는 방식
- 생성한 가상환경에
requirements.txt
를 설치하여 환경 구성 --env_manager=local
로 옵션값 지정- 실행에 있어 필요한 모든 모듈 설치 필수
python_env.yaml
파일을 이용한 virtualenv 실행일 경우- mlflow에서 제공하는 virtualenv 는
pyenv virtualenv
--env_manager=virtualenv
로 옵션값 지정- 실행에 있어 최소한으로
pyenv, mlflow
설치 필수
- mlflow에서 제공하는 virtualenv 는
- Local 환경으로 실행일 경우
# python_env.yaml
# Python version required to run the project.
python: "3.10.10"
# Dependencies required to build packages. This field is optional.
build_dependencies:
- pip
- setuptools
- wheel
# Dependencies required to run the project.
dependencies:
- mlflow==2.7.1
- torch==2.0.1
- torchtext
- scikit-learn
- pandas
2. Model Tracking 예시
3. Model Registry 및 Production 모델 선택
모델 등록 및 production 모델을 선택하는 방법은 2가지입니다.
- api code
# 가장 마지막에 실행된 run 의 실험 이름을 추출
run = mlflow.last_active_run()
experiment_id = run.info.experiment_id
experiment_name = mlflow.get_experiment(experiment_id).name
# 해당 실험에서 특정 결과지표를 기준으로 sort
df = mlflow.search_runs(
experiment_names=[experiment_name],
order_by=["metrics.f1_score DESC"],
)
# 가장 score 가 높은 run의 id 선택
best_run_id = df["run_id"][0]
# production에 사용할 models 을 추출
filter_string = "name='{}'".format(model_name)
results = client.search_model_versions(filter_string)
# 필터링된 모델들 중 best_run 일 때, production status로 변경
# 이미 production status 였던 다른 model 있었을 경우 Staging status로 변경
for res in results:
if res.run_id == best_run_id:
client.transition_model_version_stage(
name=model_name,
version=res.version,
stage="Production",
)
elif res.run_id != best_run_id and res.current_stage == "Production":
client.transition_model_version_stage(
name=model_name,
version=res.version,
stage="Staging",
)
- web ui
a. Model Tab 선택
b. 등록된 모델들 확인 가능
c. 등록된 모델에 속하는 버전들 확인 가능
4. Production 으로 선택된 모델을 BentoML 과 연동
# product_download.py
import mlflow
import bentoml
from mlflow.tracking import MlflowClient
# MLflow client 생성
client = MlflowClient()
# 모델 이름 정의
model_name = "{model_name}"
# 모델 repository 검색 및 조회
filter_string = "name='{}'".format(model_name)
results = client.search_model_versions(filter_string)
for res in results:
print(
"name={}; run_id={}; version={}; current_stage={}".format(
res.name, res.run_id, res.version, res.current_stage
)
)
# Production stage 모델 버전 선택
for res in results:
if res.current_stage == "Production":
deploy_version = res.version
# MLflow production 모델 버전 다운로드 URI 획득
model_uri = client.get_model_version_download_uri(model_name, deploy_version)
# Import logged mlflow model to BentoML for serving:
loaded_model = mlflow.sklearn.load_model(model_uri)
bento_model = bentoml.sklearn.save_model(
"sklearn_titanic",
loaded_model,
)
print("Model imported to BentoML: %s" % bento_model)
bento_model_2 = bentoml.mlflow.import_model(
"mlflow_titanic",
model_uri,
signatures={"predict": {"batchable": True}},
)
print("Model imported to BentoML: %s" % bento_model_2)
- 모델을 bentoml 형식으로 저장하는 방식은 2가지가 있습니다.
- 두 방식 중, 제공해주는
flavor
가 있는 경우 모델에 맞게 최적화가 되어 있어 파이프라인도 제공가능하므로 해당 방식을 더 선호합니다.
- 두 방식 중, 제공해주는
2. LLM 을 이용한 MLFlow
0. Background
- 앞서 sklearn의 예시와 같이 수치화가 가능한 matrix가 있을 땐, 여러 차트를 통해 시각화가 가능했습니다.
- 하지만, LLM 과 같이 성능을 측정하는데 있어서 수치화가 힘든 모델이 간혹 있기에 이번 문서에서는 측정지표로 비교하기 어려운 LLM 을 MLFlow에서 활용하는 예시에 대한 설명을 정리했습니다.
- 기본적인 동작 방식 및 UI 설명은 sklearn 과 동일합니다.
- 이 글을 작성할 당시에 사용된 특정 모듈에 대한 버전은 다음과 같습니다.
mlflow==2.7.1 bentoml==1.1.5 langchain==0.0.229 openai==0.27.8 pymilvus==2.2.8
- required files
- tracking
langchain_tracking.py
MLProject
python_env.yaml
- utils
- llms
init.py
openai.py
chat_client.py
config.py
prompt.py
- llms
requirements.txt
- tracking
1. load_chat_client
- langchain으로 사용할 model 정보가 담겨있는 client 를 반환해주는 메소드
def load_chat_client(chat_client, model_name):
qa_chain = chat_client.ready_client(
vectorstore_connection_args=openai_params.get("vector_store_info", None),
gpt_connection_args=openai_params.get("gpt_info", None),
platform_name=openai_params.get("platform_name", None),
models_args=openai_params.get("models_info", None),
service_type=openai_params.get("service_type", None),
collection_name=openai_params.get("collection_name", None),
)
return qa_chain
- 현재 mlflow - langchain 사용 시, 기능이 극히 제한적입니다.
- 현재 버전에서 QA chain 관련해서는
RetrievalQA
만 지원합니다. - 또한 langchain 사용 시, azure는 지원하지 않고 openai 만 지원합니다.
- openai 중에서도 chat-models인 gpt-3.5, gpt-4 는 공식적으로 지원하지 않습니다.
- chat-models이 아닌 llm-models만 지원
- 현재 버전에서 QA chain 관련해서는
💡따라서, 이번 실습에서는 mlflow 내부코드를 수정하여 gpt-3.5, gpt-4 등 chat-models를 사용하도록 변경하였습니다.
2. load_retriever
- mlflow에서 langchain 사용 시, retriever()를 return 으로 던져주는 메소드가 필요
def load_retriever(persist_dir):
embeddings = OpenAIEmbeddings(
model=config.EMBEDDING_MODEL,
openai_api_key=config.OPENAI_API_KEY,
)
vectorstore = Milvus(
embeddings,
connection_args={
"host": config.MILVUS_HOST,
"port": config.MILVUS_PORT,
"user": config.MILVUS_USER,
"password": config.MILVUS_PASSWORD,
},
collection_name=config.COLLECTION_NAME,
)
return vectorstore.as_retriever()
3. Model runs
- gpt-3.5, gpt-4 두 모델을 비교해보는 테스트 코드 작성예시
if __name__ == "__main__":
run_ids = []
artifact_paths = []
model_names = [config.GPT35_MODEL, config.GPT4_MODEL]
chat_client_35 = ChatClient()
chat_client_4 = ChatClient()
openai_35 = load_chat_client(chat_client_35, model_names[0])
openai_4 = load_chat_client(chat_client_4, model_names[1])
client = MlflowClient()
mlflow.set_tracking_uri(config.MLFLOW_TRACKING_URI)
for model, name in zip([openai_35, openai_4], model_names):
with mlflow.start_run():
run = mlflow.last_active_run()
artifact_path = f"models/{name}"
lc_model = model
# Log the model as an artifact of the MLflow run.
print("\nLogging the trained model as a run artifact...")
mlflow.log_param("platform", config.PLATFORM_NAME)
mlflow.log_param("collection name", config.COLLECTION_NAME)
mlflow.log_param("embedding model name", config.EMBEDDING_MODEL)
logged_model = mlflow.langchain.log_model(
lc_model=lc_model,
loader_fn=load_retriever,
artifact_path=artifact_path,
registered_model_name=name,
)
print("Model uri:", logged_model.model_uri)
run_ids.append(mlflow.active_run().info.run_id)
artifact_paths.append(artifact_path)
client.update_run(run.info.run_id, "FINISHED", f"log_model_{name}")
print(
"\nThe model is logged at:\n%s"
% os.path.join(mlflow.get_artifact_uri(), name)
)
- 앞서 정의했던
ChatClient()
객체를 모델 별 생성합니다. - for-loop 으로 테스트를 진행할 model의 수 만큼 iteration 을 실행합니다.
- 각 iter 마다 저장할 파라미터들을
log_param
으로 지정합니다. mlflow.langchain.log_model
메소드를 사용하여 다음과 같은 파라미터를 지정합니다.lc_model
: 저장할 llm model- 여기선
RetrievalQA
- 여기선
loader_fn
: retriever 를 return 해주는 메소드artficat_path
: 저장할 artifact pathregistered_model_name
: 저장할 모델 이름
- 다음 단계에서 진행할 llm prompt evaluate 를 위해
run_ids
,artifact_paths
에 해당하는 값들을 append 합니다.
4. LLM Prompt Evaluate
for i in range(len(model_names)):
with mlflow.start_run(run_id=run_ids[i]):
eval_df = pd.DataFrame(
{
"query": [
"Q: 객체가 뭐에요?",
"Q: 객체지향이란?",
"Q: 비전이 뭐에요??",
"Q: 퇴근할 수 있는 가장 좋은 방법은?",
]
}
)
print(eval_df)
evaluation_results = mlflow.evaluate(
model=f"runs:/{run_ids[i]}/{artifact_paths[i]}",
model_type="text",
data=eval_df,
)
- 모델 및 각각 실행된 run 들에 대하여 비교가 가능합니다.
- 앞서 gpt-3.5, gpt-4 에 대한 runs 들의 id 값을 저장한 run_ids 를 사용합니다.
- for-loop 을 iteration을 하면서 각 run_id에 대하여 주어진 eval_df인 query 들을 사용하여 추론합니다.
- 각각의 결과는 run에 해당하는 evaluation에 저장됩니다.
5. Model Tracking 예시
이렇게 수치화가 가능한 matrix 를 통해 모델 성능을 평가하는 방식과 수치화가 가능한 matrix가 마땅치 않은 llm 에서 사용하는 방식을 정리해봤습니다.
이렇게 작성하고 보니 확실히 모델 성능을 테스트 하는 상황이나 모델을 배포하는 상황 등 다양한 상황에서 사용하기에 좋다라고 생각합니다.
이번에 작성드린 사용예시는 정말 간단한 사용방법만 정리해서 올린 것이기에 실무에서 적용하려면 다양한 추가 기능들을 살펴보며 구현해보시길 권장드립니다!!
다음으로는 실험이 끝난 뒤 실제 배포를 위한 모델이 선택되었을 때,
사용 할 수 있는 bentoml에 대해 알아보도록 하겠습니다!
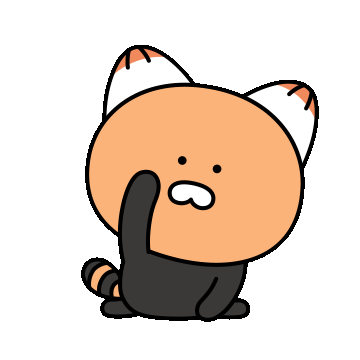
Reference